Data Science and AI Bootcamp
- Online
- 4 Months
- 17th February
- Tech Classes: Monday-Thursday 7:00 PM-9:00 PM (PKT)
- Professional Development: Friday 7:00 PM-9:00 PM (PKT)
- Industry Expert Sessions: Saturday 12:00 PM-2:00 PM (PKT)
Course Description
This four-month-long Data Science and AI Bootcamp is designed to equip you with essential Data Science and AI skills like Business Intelligence, Machine Learning, Deep Learning, Large Language Models (LLMs), NLP, Computer Vision, Generative AI, and more.
This program will teach you Python for Data Analytics, a key skill for anyone looking to work in data science and AI. You’ll learn how to create and manage data pipelines, work with multimodal data like text and images, and develop AI agents for practical use. The course also covers essential areas like Natural Language Processing (NLP), Computer Vision (CV), and Machine Learning Operations (MLOps). By the end, you’ll have the knowledge and confidence to start a successful career in Data Science and Artificial Intelligence, whether locally or internationally.
Join us on this journey and become a future-ready in Data Science and AI.
Course Curriculum
Python Basics
- Introduction to Python: history, features, and advantages
- Expressions and operators: arithmetic, assignment, comparison, and logical
- Understanding type() function and type inference
- Introduction to data structures: lists, tuples, and dictionaries
- Working with arithmetic operators: addition, subtraction, multiplication, division, modulus, and exponentiation
- Using comparison operators: equal to, not equal to, greater than, less than, etc.
- Logical operators: and, or, and not
- Exploring advanced data types: sets and strings manipulation.
Expressions, Conditional Statements & For Loop
- Evaluating expressions: operator precedence and associativity
- Introduction to conditional statements: if, elif, and else
- Executing code based on conditionals.
- Understanding the flow of control in conditional statements
- Iteration using the for loop: range(), iteration over lists, and strings.
While loop, Break and Continue Statements, and Nested Loops
- Working with while loop: syntax, conditions, and examples
- Combining loops and conditionals
- Using the break statement to exit loops prematurely.
- Utilizing the continue statement to skip iterations.
- Implementing nested loops for complex iterations
Functions
- Introduction to functions: purpose, advantages, and best practices
- Defining and calling user-defined functions
- Parameters and arguments: positional, keyword, and default values
- Return statement and function output.
- Variable scope and lifetime
- Function documentation and code readability
Exception Handling and File Handling
- Understanding exceptions: errors, exceptions, and exception hierarchy
- Handling exceptions using try-except blocks: handling specific exceptions, multiple exceptions, and else and finally clauses.
- Raising exceptions and creating custom exception classes
- File handling in Python: opening, reading, writing, and closing files.
- Working with different file modes and file objects
Mathematical Concepts
Linear Algebra:
- Vectors, matrices, operations, and their applications in data science.
- Introduction to numpy for matrix operations.
Introduction to numpy:
- Why numpy is the preferred library for numerical operations in Python.
- Basic numpy operations: creating arrays, array indexing, array slicing.
- Matrix operations using numpy: matrix multiplication, finding determinants, solving linear equations.
Probability and Statistics
Probability Theory:
- Basic probability, rules, and distributions (normal, binomial, Poisson).
- Random variables, expectations, and variance.
- Measures of central tendency (mean, median, mode).
- Measures of variability (range, variance, standard deviation)
- Using pandas for data manipulation and summary statistics.
- scipy for performing hypothesis tests and building confidence intervals.
- Visualization with seaborn and matplotlib to understand data distributions and relationships.
Probability and Statistics II
Inferential Statistics:
- Sampling distributions and the Central Limit Theorem.
- Confidence intervals and hypothesis testing.
Correlation and Regression:
- Scatter plots, correlation coefficients.
- Simple linear regression analysis.
- Visualization of correlation matrices and scatter plots to identify relationships between variables.
- Advanced plotting techniques such as pair plots, heatmaps, and regression plots to extract deeper insights from data.
Introduction and Missing Value Analysis
- Introduction to Exploratory Data Analysis (EDA)
- Importance of EDA in data analysis
- Steps involved in EDA
- Handling missing values: identification, analysis, and treatment strategies • Imputation techniques for missing values
Data Consistency, Binning, and Outlier Analysis
- Data consistency checks using fuzzy logic
- Binning and discretization techniques for continuous variables
- Outlier detection and analysis methods
- Handling outliers: techniques for treatment or removal
Feature Selection and Data Wrangling
- Importance of feature selection in EDA
- Feature selection techniques: filter methods, wrapper methods, and embedded methods
- Data wrangling: cleaning and transforming data for analysis
- Handling categorical variables: encoding techniques
Inference, Hypothesis Testing, and Visualization
- Inference and hypothesis testing in EDA
- Common statistical tests: t-test, chi-square test, ANOVA, etc.
- Visualization techniques for EDA: histograms, box plots, scatter plots, etc.
- Hands-on practical session for complete EDA using a dataset
Machine Learning and Deep Learning
Overview of Machine Learning
- Supervised, unsupervised, and reinforcement learning.
- Machine learning workflow from data collection to model deployment.
- Introduction to Python libraries essential for ML (Scikit-learn, TensorFlow, PyTorch)
Supervised learning and Linear Regression
- Linear Regression: Concept, loss function, evaluation metrics (MSE, R²)
- Building a Linear Regression model in scikit-learn
- Visualizing predictions vs actuals
Machine Learning Performance Metrics and Naive Bayes
- Evaluation metrics for classification problems: accuracy, precision, recall, F1 score, etc.
- Introduction to Naive Bayes algorithm and its applications
- Implementing Naive Bayes for classification tasks
Logistic Regression, SVM, Decision Trees, and Random Forests
- Logistic Regression: theory, interpretation, and applications
- Support Vector Machines (SVM): concepts, kernels, and use cases
- Decision Trees: construction, pruning, and interpretability
- Random Forests: ensemble learning and feature importance
- Bagging and Boosting: techniques for improving model performance
Clustering Introduction, Partitioning Algorithms, and Cluster Evaluation
- Introduction to clustering: unsupervised learning technique
- Partitioning algorithms: K-means, K-medoids
- Hierarchical clustering: agglomerative and divisive approaches
- Density-based clustering: DBSCAN, OPTICS
- Cluster evaluation metrics: silhouette coefficient, Davies-Bouldin index
Regression and Evaluation of Regression Methods
- Introduction to regression analysis
- Linear regression: assumptions, interpretation, and model evaluation • Evaluation metrics for regression: mean squared error, R-squared, etc.
- Other regression methods: polynomial regression, ridge regression, lasso regression
Introduction to Deep Learning
- Overview of deep learning, its importance in computer vision, key concepts, and architectures.
- Code along session for building Deep Neural Network from scratch
Deep Learning Hyperparameter Tuning
- Strategies for optimizing hyperparameters like learning rate, batch size, and regularization to improve model performance.
Conceptual Overview
- Understand the distinct roles and responsibilities of data scientists and data engineers within projects.
Data Sourcing with Python
- Extracting data from sources like PostgreSQL, MongoDB, APIs, web scraping, and IoT devices.
Databricks Tour
- Get introduced to Databricks as a platform for big data processing and machine learning.
Big Data Handling for Data Science
- Process large-scale datasets using Apache Spark within a distributed environment.
MLflow
- Learn to track experiments and ensure reproducibility in machine learning workflows
Introduction to Convolutional Neural Networks (CNNs)
- Explanation of CNNs, their architecture, and their role in image processing.
- Codel along session on Convolutional Neural Networks.
Building Custom Image Classification Models
- Step-by-step guide to creating and training a custom image classifier using a CNN.
Transfer Learning and Introduction to Object Detection
- Introduction to transfer learning, its applications, and an overview of object detection techniques.
- Hands on with YOLO Object Detection
- Practical session on using the YOLO (You Only Look Once) algorithm for object detection.
Custom Training YOLO model
- Detailed guidance on training a YOLO model with a custom dataset for specific object detection tasks.
Using State-of-the-Art Models for Real-World Applications
- Exploring and implementing advanced models in computer vision for practical use cases.
Introduction to OpenCV
- Introduction to OpenCV, its libraries, and its importance in computer vision tasks.
Image Pre-processing and Pre-build Algorithms in OpenCV
- Hands-on session on image pre-processing techniques and using built-in algorithms in OpenCV.
Advance guided project with OpenCV
- Capstone project where Students apply learned techniques in a guided project using OpenCV.
Introduction to NLP and Text Normalization
- Overview of Natural Language Processing (NLP)
- Techniques for text normalization: lowercasing, punctuation removal, etc.
- Basics of tokenization and stopword removal
Text Representation and Tokenization
- Introduction to vectors in NLP: Bag of Words and Count Vectorizer
- Basics of tokenization and stopword removal
Stemming, Lemmatization, and N-gram Language Models
- Understanding and applying stemming and lemmatization
- Introduction to N-gram language models
- Introduction to vectors in NLP: Bag of Words, Count Vectorizer, and TF-IDF
Markov Models and Language Model Evaluation
- Basics of Markov models in NLP
- Overview of Text Classification
- Techniques for evaluating language models: probability smoothing and performance metrics
- Introduction to Naive Bayes and Sentiment Classification
Advanced Classifiers and Vector Semantics
- Generative vs. discriminative classifiers
- Understanding vector semantics and embeddings
- Introduction to neural word embeddings: Word2Vec and GloVe
Deep Learning for NLP and Sequence Models
- Overview of deep learning techniques for NLP
- Applying supervised and unsupervised learning methods to NLP tasks
- Exploring sequence of words in NLP tasks
Transformers and Large Language Models
- Understanding the architecture and mechanisms of transformers
- Overview of large language models (LLMs) and their capabilities
- Hands-on: Fine-tuning transformers and LLMs for NLP applications
Training and Fine-Tuning LLMs
- Techniques for training large language models
- Fine-tuning pre-trained LLMs for specific tasks
Multimodal Integration
- Exploring multimodal models and their integration
- Working with open-source models for diverse applications
- Utilizing cloud APIs for deploying and integrating multimodal solutions
LLM Agents
- Introduction to LLM agents
- Use cases and applications of LLM agents
- Techniques for designing and deploying LLM agents for various tasks
Introduction to Generative AI and Multimodal Models
- Overview of Generative AI and its impact on various industries.
- Understanding multimodal models and how they differ from single-modality models.
- Exploring the OpenAI API: Capabilities and applications for text, speech, and image-based AI.
Speech Synthesis and Conversational Agents
- Objective: Dive into text-to-speech (TTS) and speech-to-text (STT) models and explore conversational AI with agent frameworks.
- Overview of text and voice synthesis technologies.
- Introduction to Eleven Labs and Heygen: Creating high-quality audio and speech synthesis.
- Using OpenAI API for speech synthesis and understanding its capabilities
Advanced Agent Development with LangChain
- Basics of LangChain and its role in agent-based AI.
- Building, customizing, and deploying agents using LangChain.
- Exploring integration of LangChain with other APIs for multimodal interactions, including OpenAI API for image and text generation.
Building Complex Agent-Based Applications with CrewaI, Synthesia, and OpenAI
- Introduction to CrewaI: Building intelligent agents for various tasks.
- Synthesia for video and visual content generation in AI-driven applications.
- Using OpenAI API to generate multimodal content (text, images, and speech).
- Building a final project: Integrate multiple APIs to create a multimodal generative application.
Introduction to MLOps and AI/NLP Fundamentals
- Overview of MLOps and its importance in the AI lifecycle
- Current trends in AI
- Setting up the development environment
Deep Dive into Machine Learning Models for NLP
- Understanding NLP models (llama2, GPT, Mistral, etc.)
- Introduction to Hugging Face Transformers and Datasets
- Hands-on: Building a simple NLP model with Hugging Face
Introduction to FastAPI for ML Model Deployment
- Basics of API development with FastAPI
- Deploying a simple ML model with FastAPI
- Hands-on: Creating your first ML API with FastAPI
Advanced FastAPI Features for Production-Ready APIs
- Authentication and authorization in FastAPI
- Hands-on: Enhancing your ML API with advanced features
Docker Image Creation and Application Containerization
- Introduction to Docker and its role in application deployment
- Hands-on: Creating Docker images for NLP applications
- Converting existing applications into Docker containers for scalable deployment
LangGraph and Advanced Model Integration
- Introduction to LangGraph for building graph-based NLP Applications
- Exploring advanced integration scenarios with LangGraph and LangChain
- Hands-on: Designing graph-based workflows for NLP tasks
Deploying ML Models on Google Cloud
- Overview of Google Cloud Platform (GCP) for ML
- Introduction to Google Cloud Run
- Hands-on: Deploying your Dockerized FastAPI application on GCP with LangSmith monitoring
STANDARD MONTHLY
-
For 4 Months
LUMPSUM
-
For 4 Months
Check your Eligibility
Currently enrolled or have graduated with a bachelor’s degree in:
- Computer Science or related fields
- Any Engineering Discipline (Mechanical, Electrical, Civil etc)
Complete our Bootcamp and Become Job Ready
We help our successful graduates find Jobs and Internships, and also provide guidance in discovering Freelance opportunities.
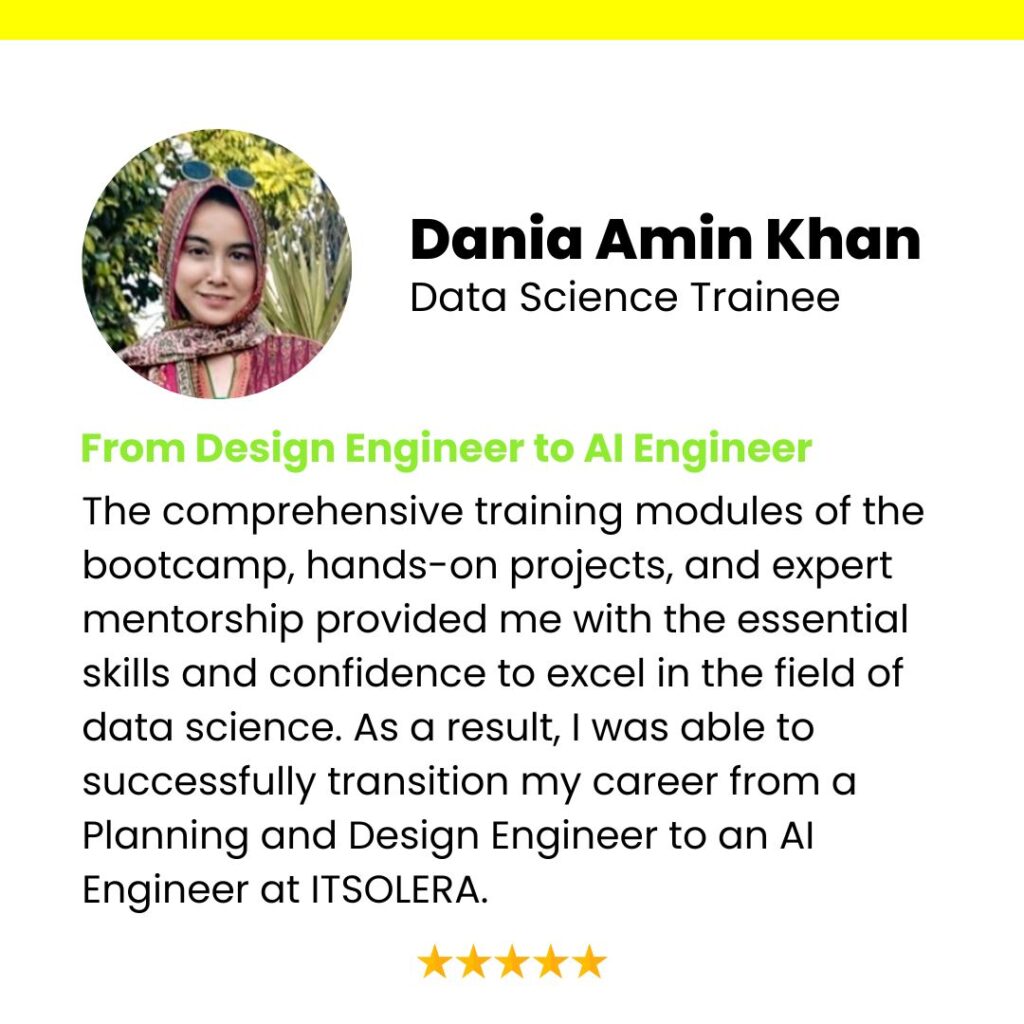
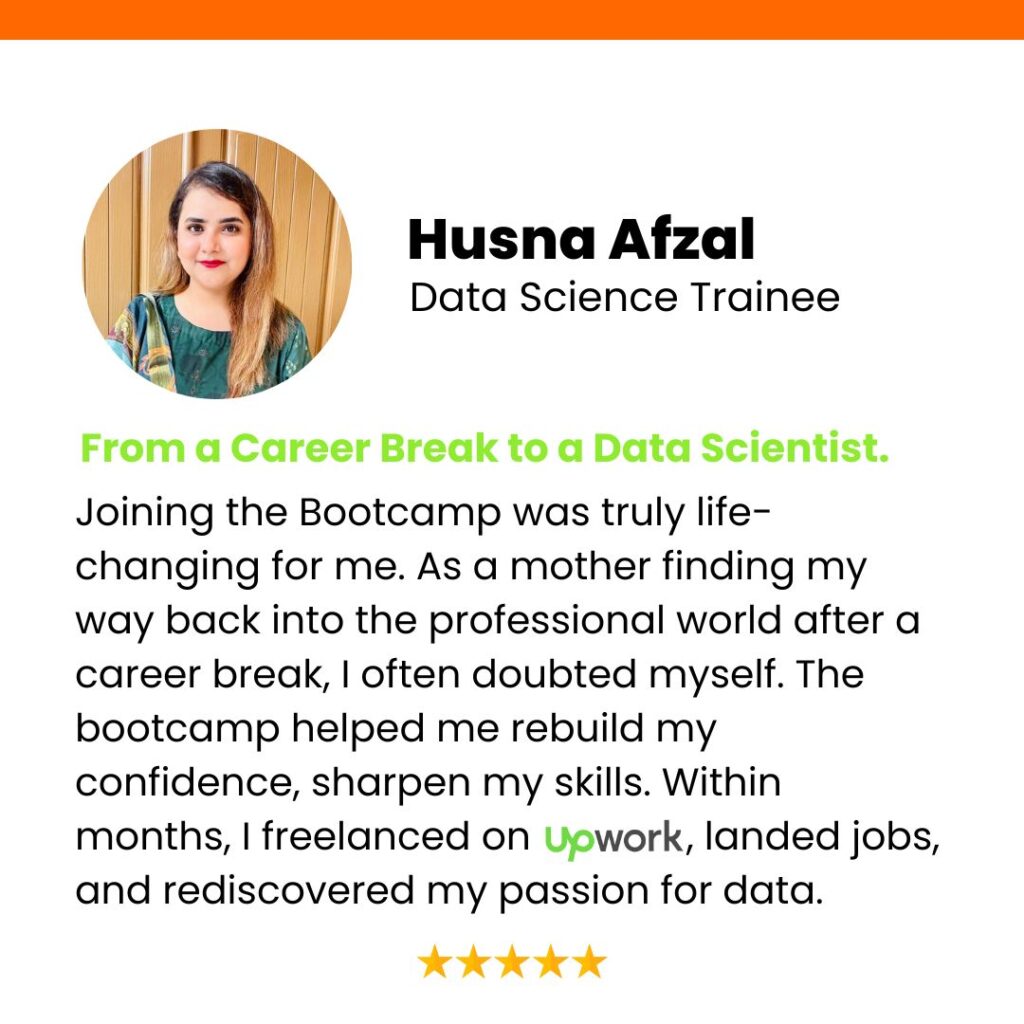
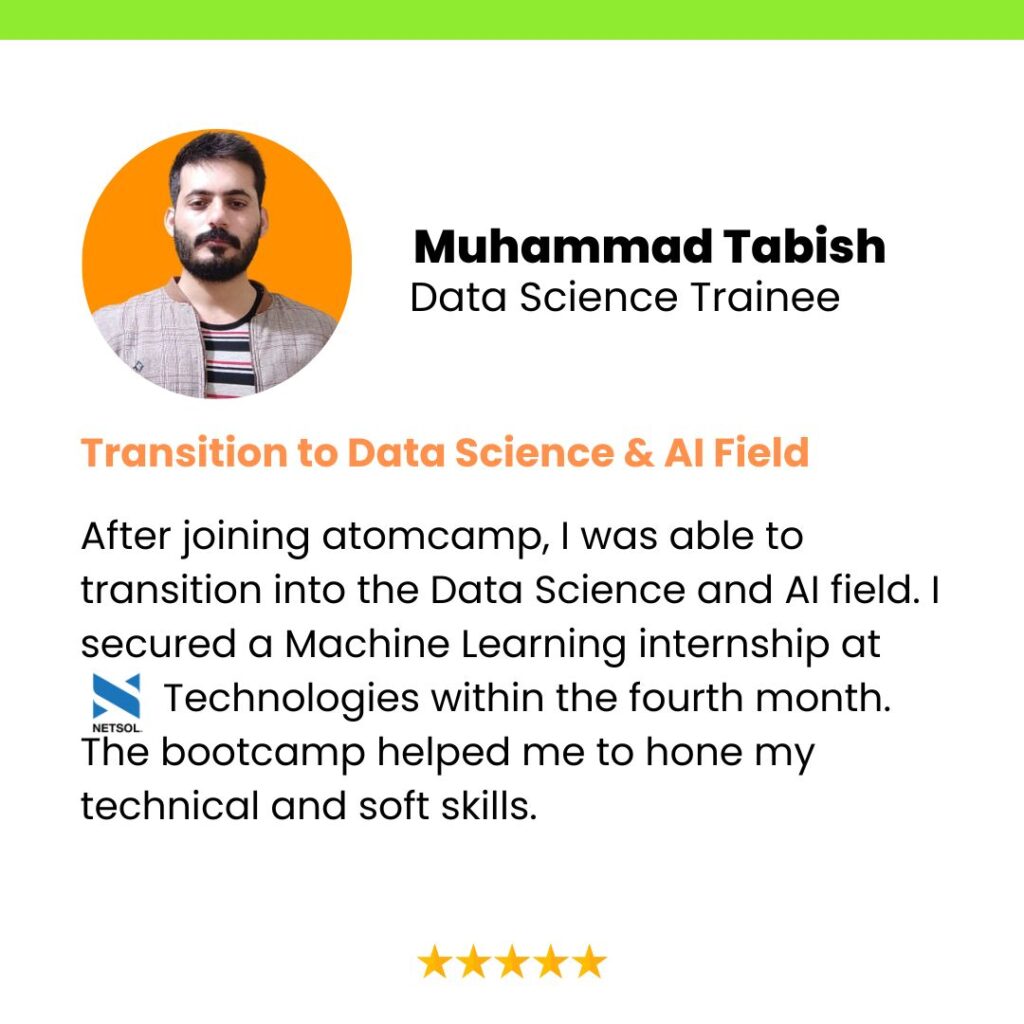
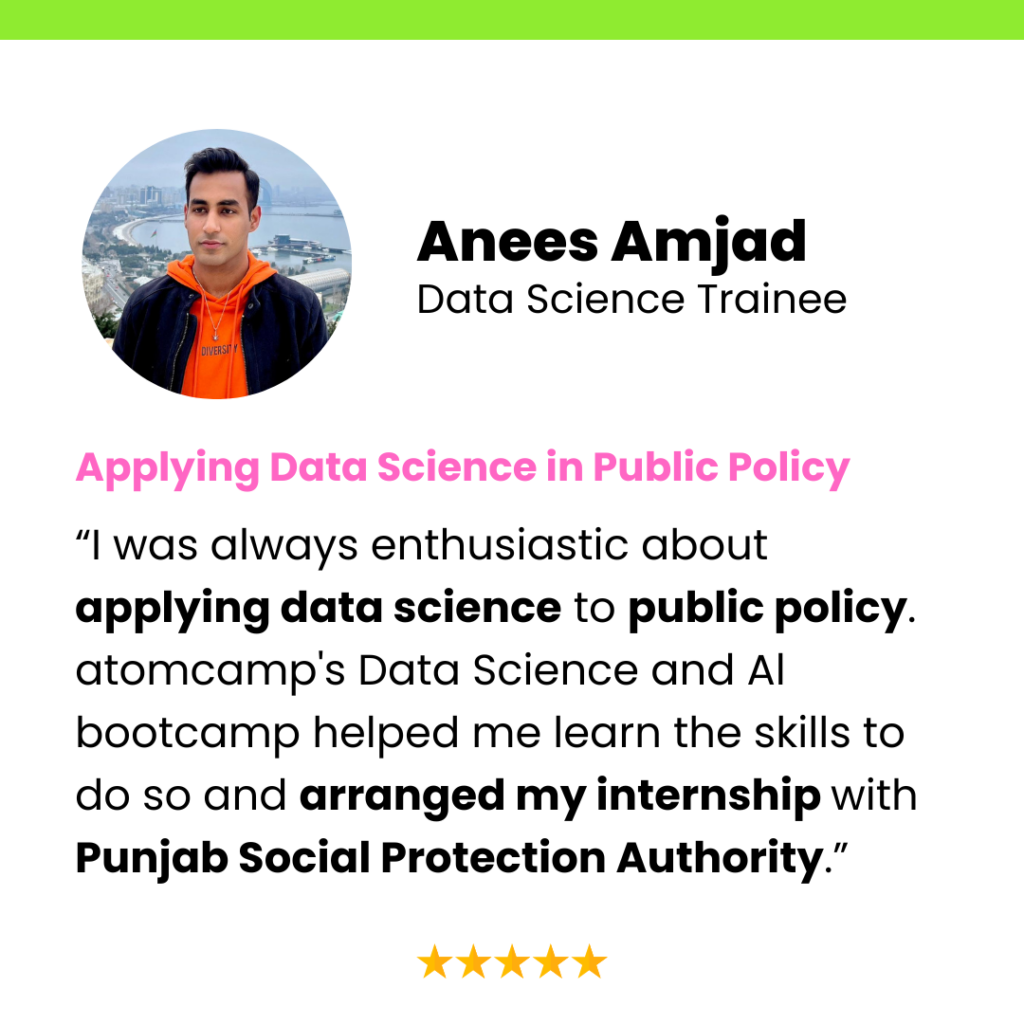
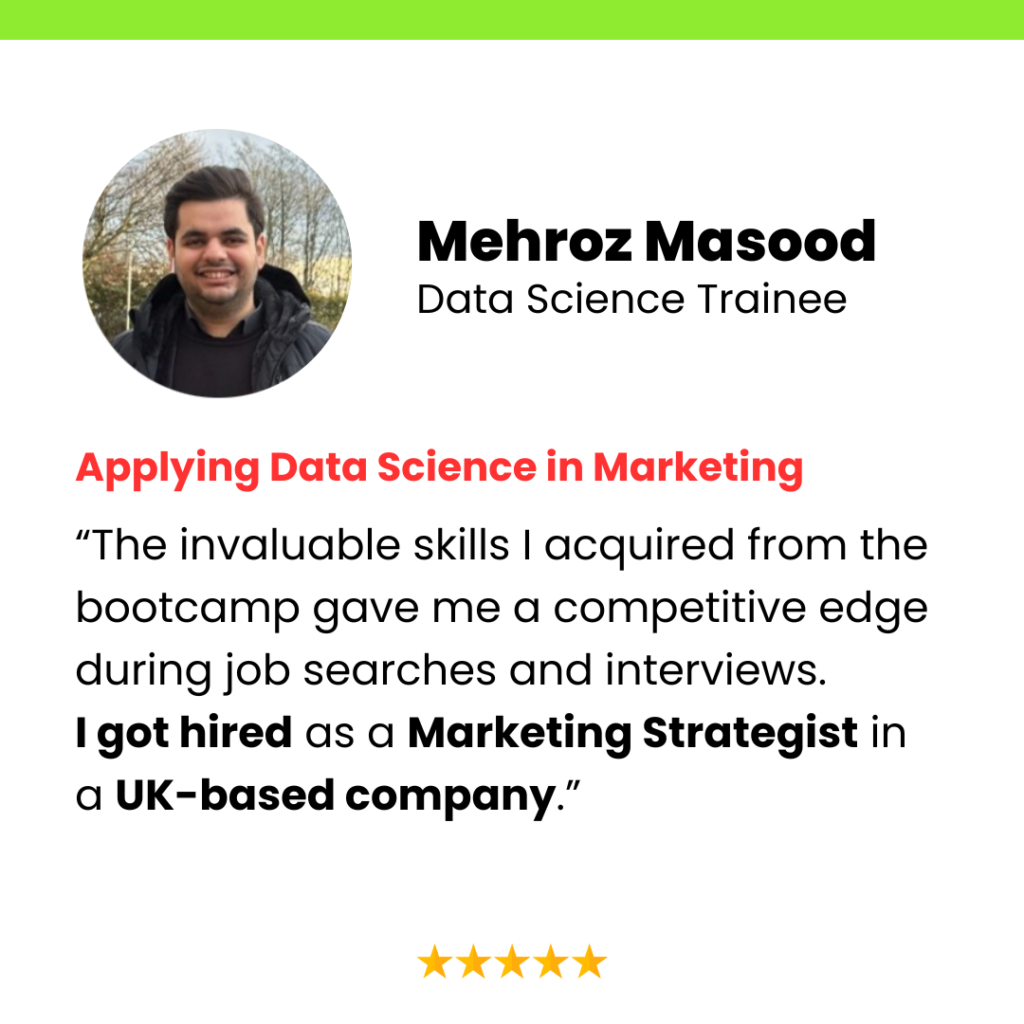
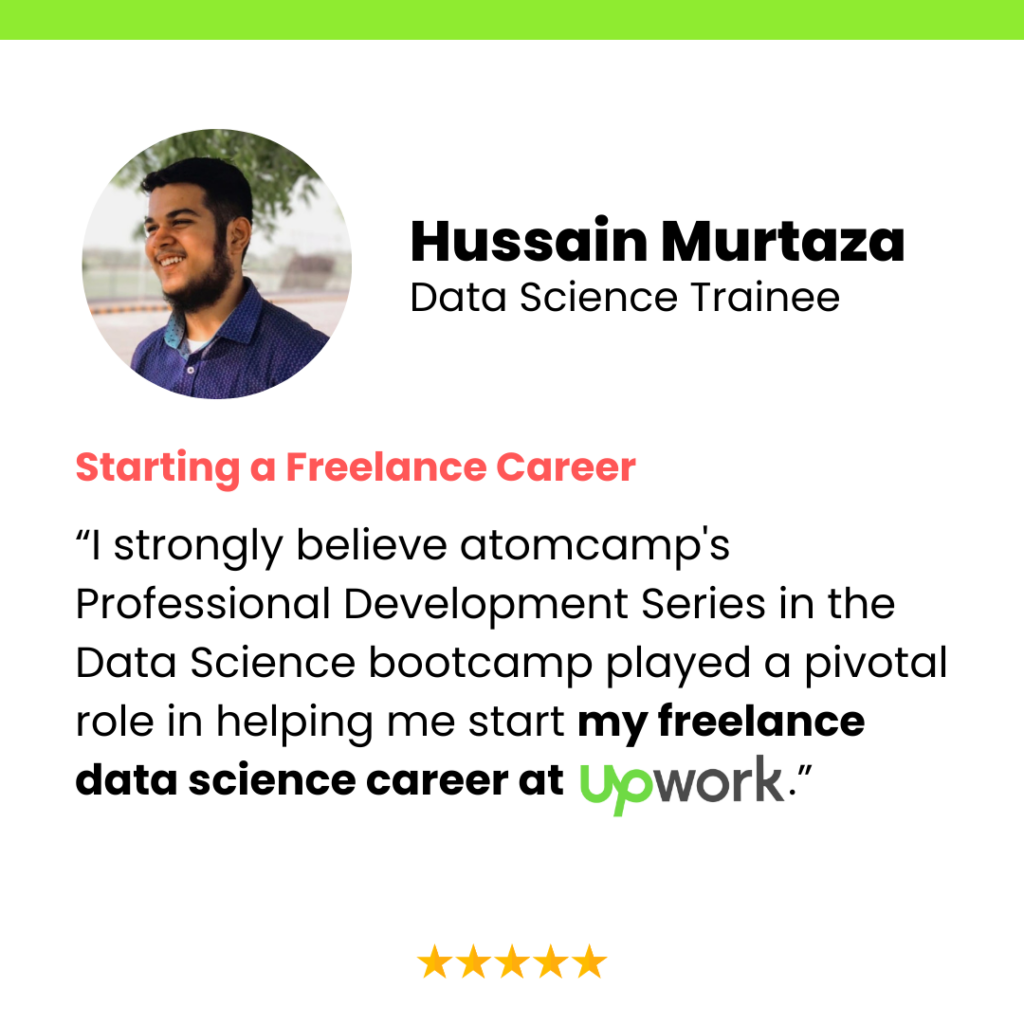
Not Sure How We Teach? Take a Demo Class.
Join a free demo class and see how we make learning simple and fun!
Key Features
Industry Ready Portfolio
Craft a portfolio that showcases your industry-ready data science projects.
Enhance your career by learning Data Science and AI skills.
Strengthen your data skills and excel in your existing career.
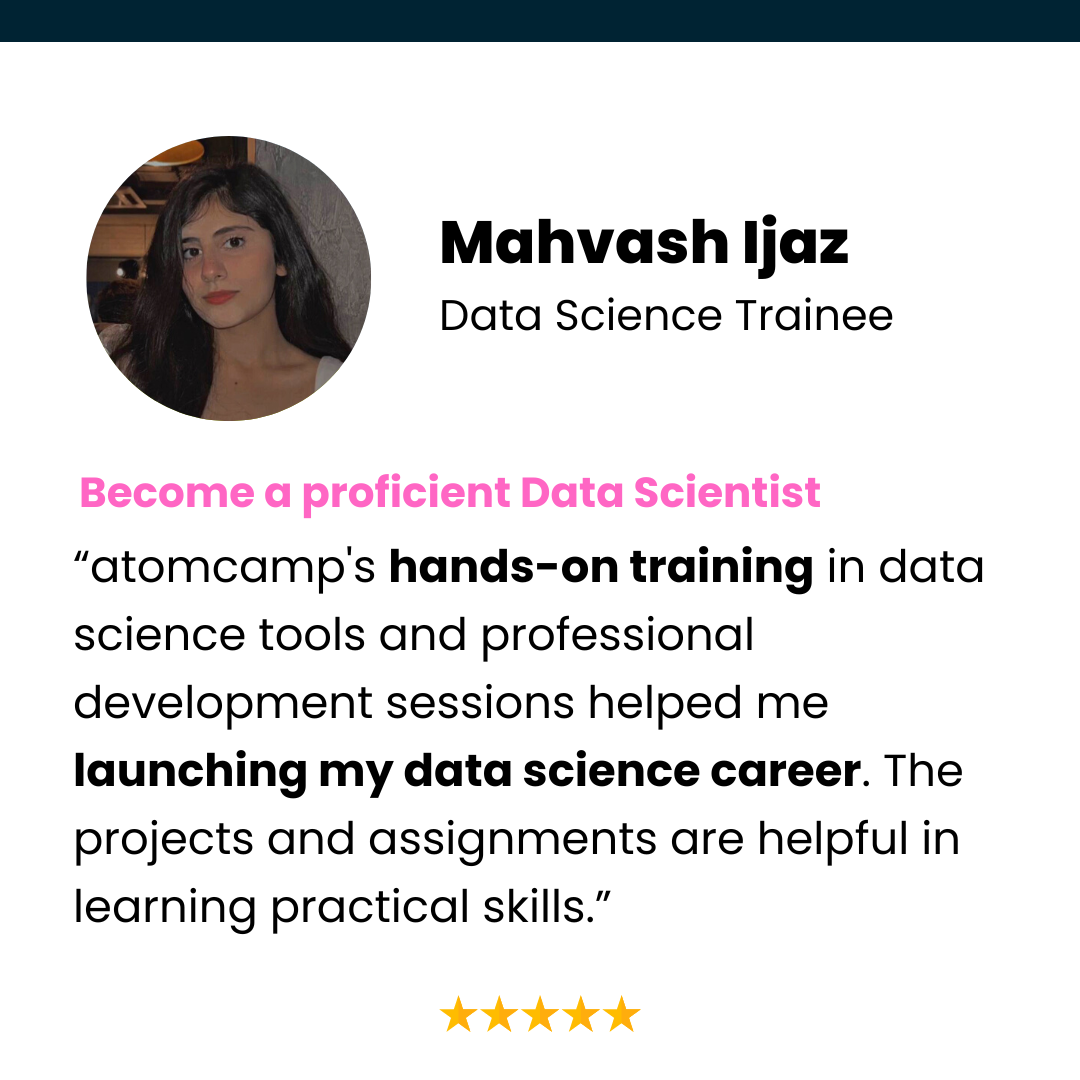
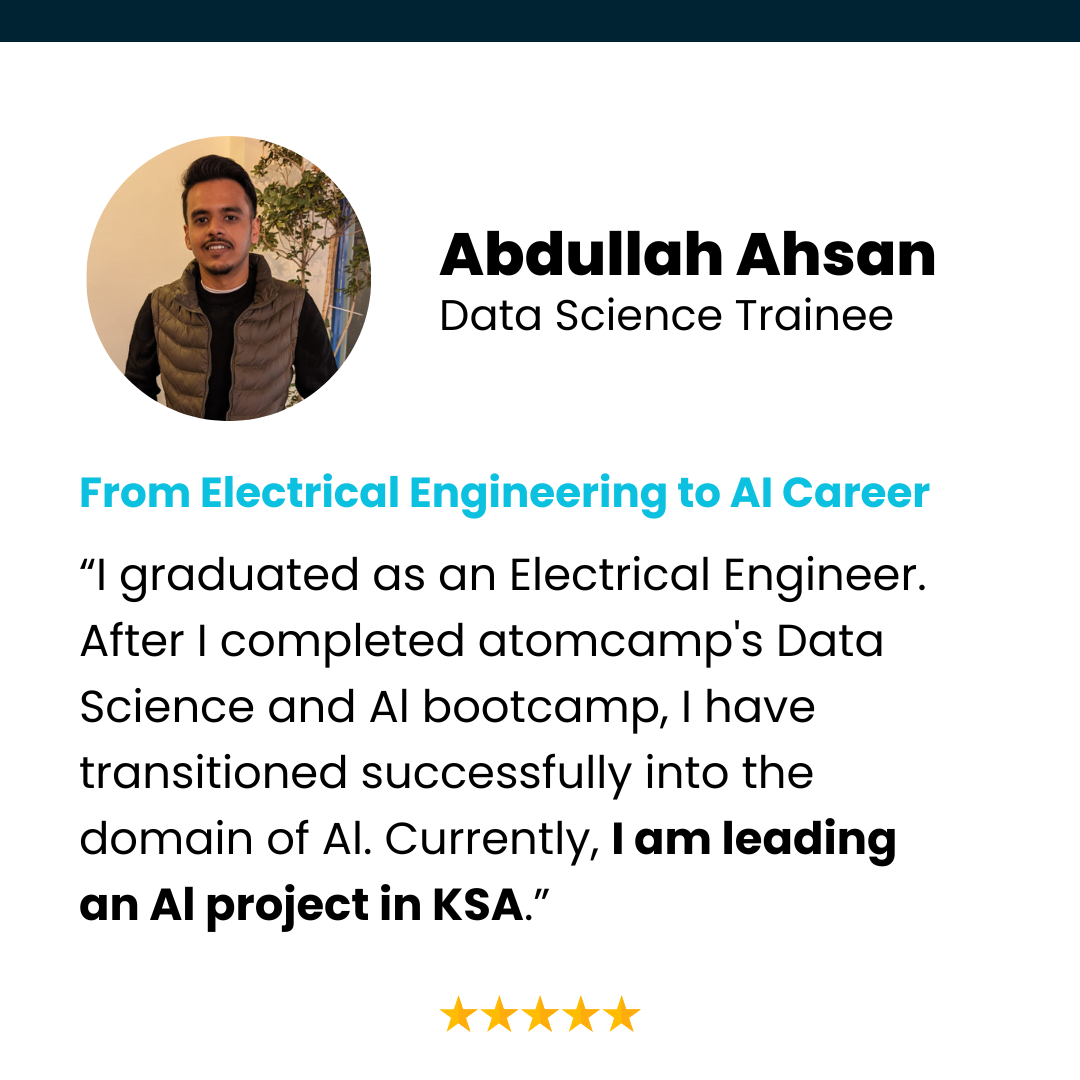
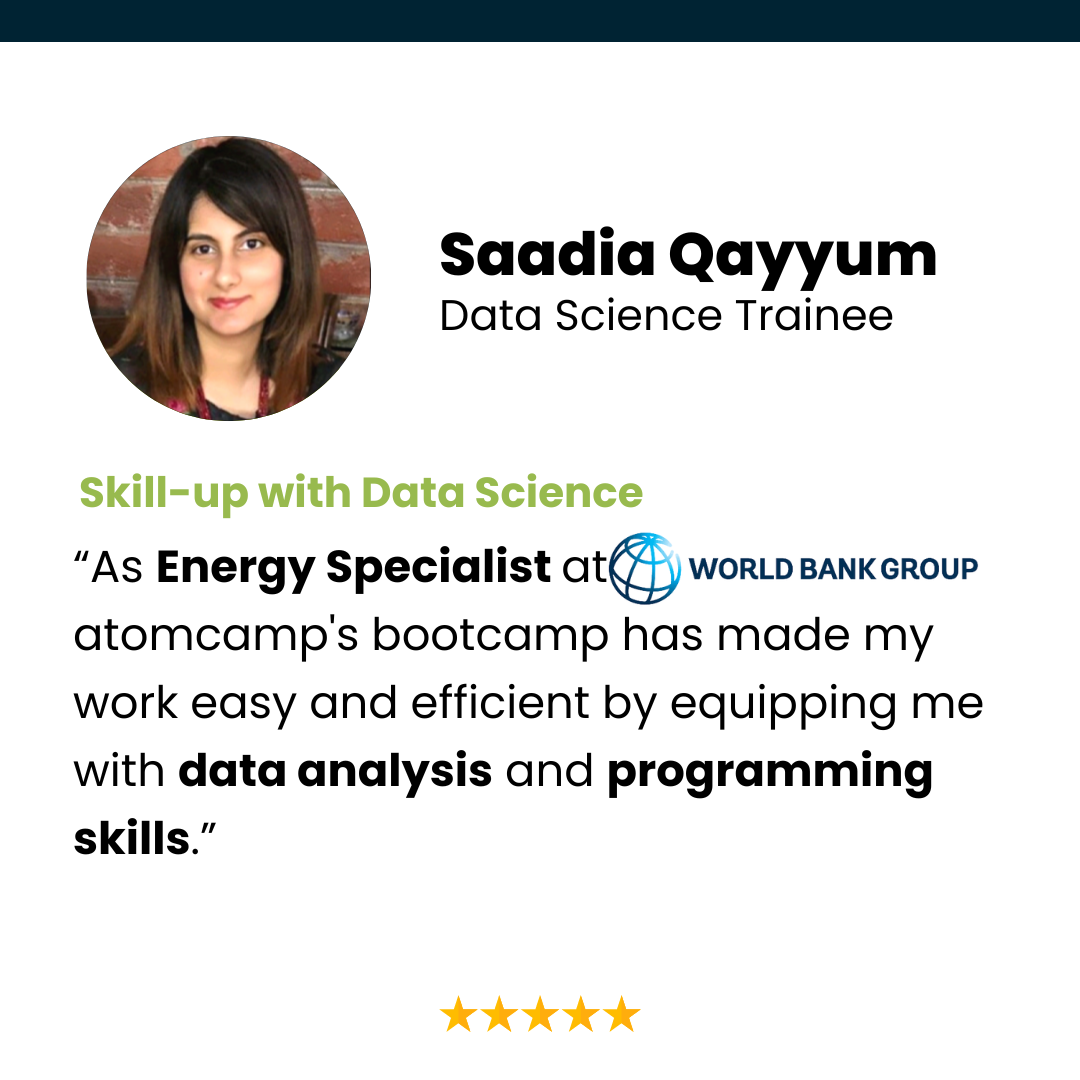
Earn a Verified Certificate of Completion
Earn a data science certificate, verifying your skills. Step into the market with a proven and trusted skillset.
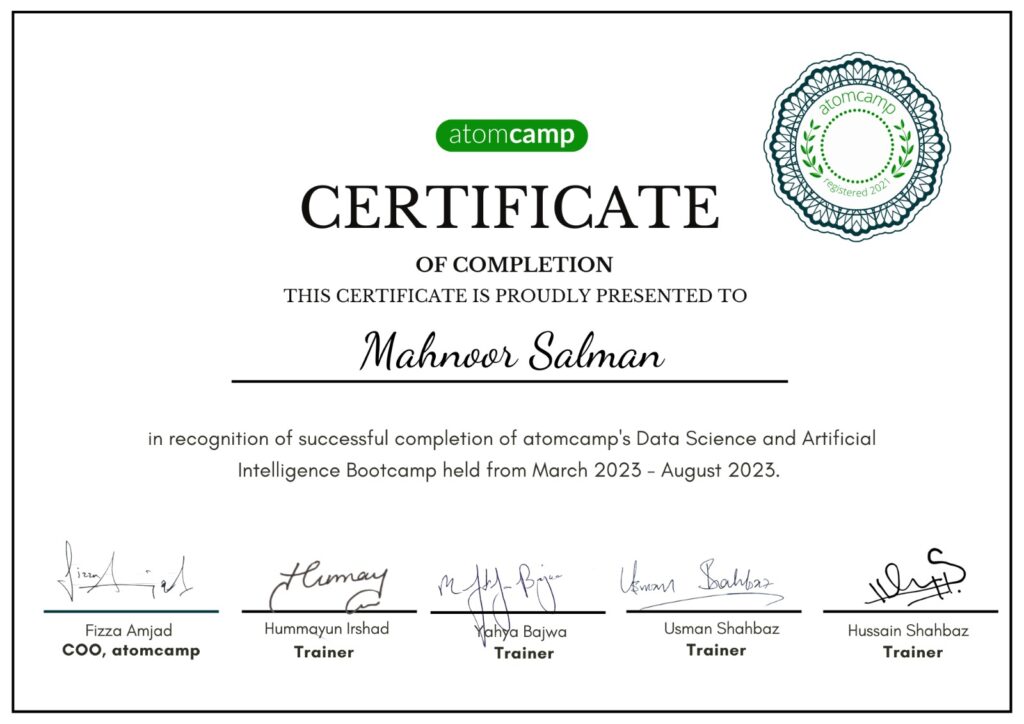
Potential Job Route
Machine Learning Engineer
The average salary of a machine learning engineer in the US is $170,000.
Artificial Intelligence Engineer
The average salary of a AI engineer in the US is $1,20,000.
Data Scientist
The average salary of a data science engineer in the US is upto $200,000.
Trusted By Leading Companies
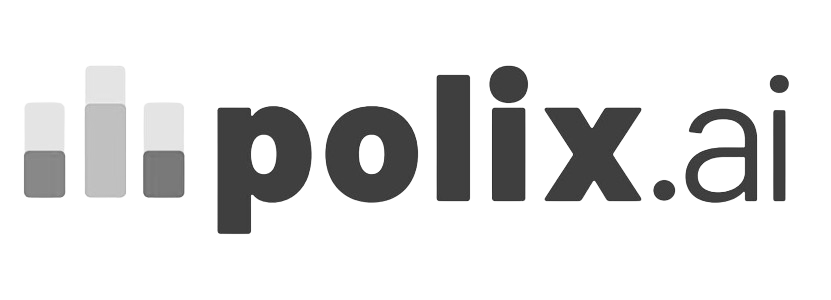
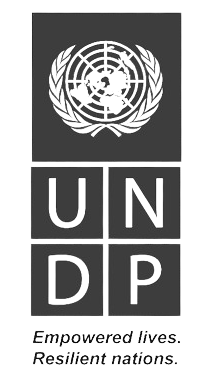
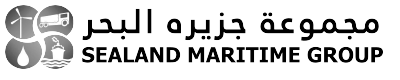
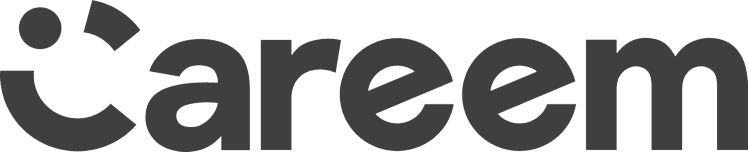
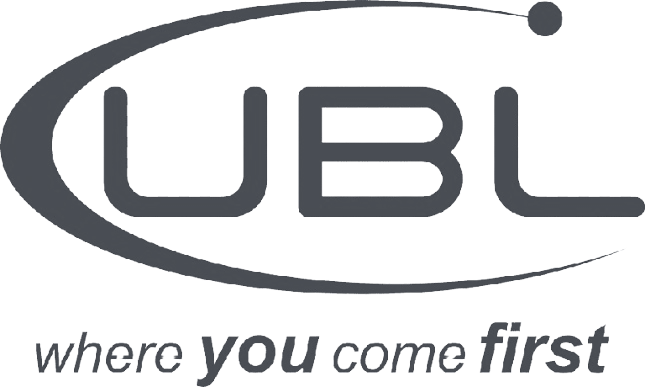
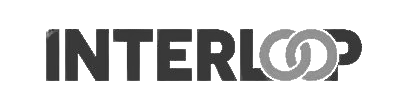
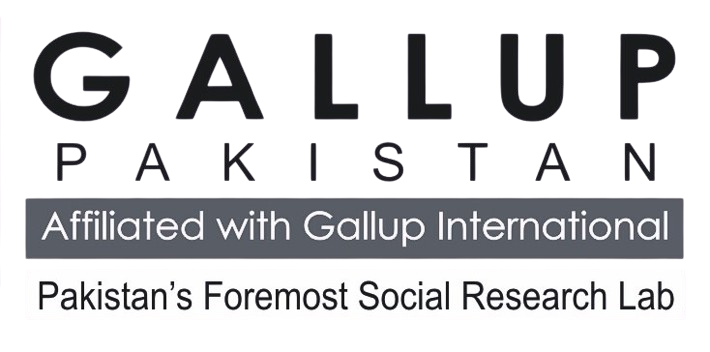
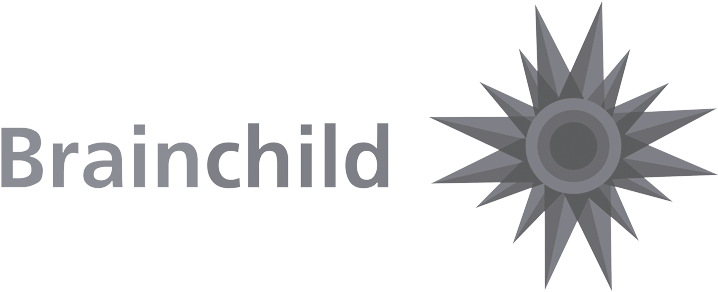
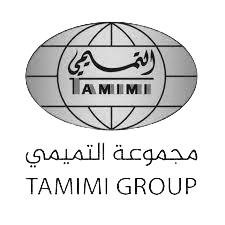
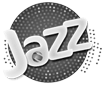
Pricing Plan
STANDARD MONTHLY
-
Standard Monthly
-
For 4 Months
LUMPSUM PAYMENT
-
Lumpsum (Advance Payment)
-
For 4 Months
WOMEN DISCOUNT
-
Standard Monthly: 25,000/per month
-
For 4 Months
Husna Afzal
Eesha Arshad Khan
Sadeen Farid
Our Guest Speakers
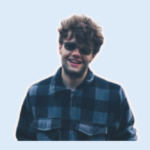
Allen Roush
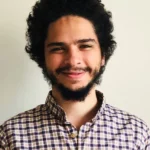
Yousaf Husni
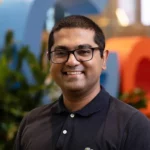
Saad Hamid
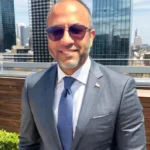
Adnan Hashmi
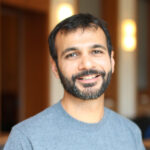
Jehangir Amjad
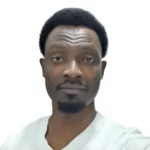
Moshood Yahaya
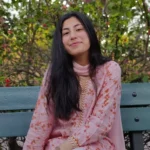
Mominah Asif
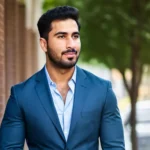
Zain ul Hassan
Frequently Asked Questions
Classes run five days a week, ensuring an immersive and engaging learning experience.
Our bootcamp explores cutting-edge data science technologies, covering machine learning, data analysis, and essential programming languages.
Absolutely! Active participation is crucial, with 80% attendance required for certification.
Yes, participants dive into hands-on assignments, reinforcing learning and practical application.
Definitely! We actively support participants in securing internships or jobs, rewarding those who show exceptional commitment and engagement.
A Bachelor’s Degree in various fields is accepted, including Computer Science, Engineering, Maths, Stats, Economics, and Business
We recommend investing 2 extra hours daily to dive into materials, ace assignments, and truly embrace the learning journey.
Our bootcamp features live training sessions, providing real-time interaction with instructors and peers.
Fulfill attendance, engage actively, complete assignments, and demonstrate proficiency in the taught skills.
Regular attendance is encouraged, but we understand life happens. Communicate any issues, and we'll support your journey.
Yes, we host Teaching Assistant (TA) sessions for reviewing material, clarifying concepts, and addressing queries.
Fill out the application form below, and our admissions team will guide you through the next steps.
It covers essential math concepts, laying the groundwork for understanding and applying advanced data science and machine learning algorithms.
This series focuses on soft skills, industry awareness, and career readiness, ensuring you're well-equipped for success in data science.